Research
Locomotion in real-world environments is particularly challenging for robots due to the unpredictable and dynamic nature of these settings. Unlike controlled laboratory conditions, real-world terrains vary in texture, slope, and stability, requiring robots to navigate uneven surfaces, obstacles, and changes in elevation. Environmental factors such as weather, debris, and interactions with other entities add further complexity. Stability and reliability in robotic locomotion require robust fault tolerance, as events like lesions, perturbations, and sensor failures are inevitable and significantly impact locomotion. While animals exhibit remarkable robustness to such disruptions, allowing them to recover and continue their tasks, robots must achieve similar resilience, especially in high-stakes scenarios like search and rescue. My research, inspired by the robustness seen in animals, investigates enhancing robot locomotion through sensory-motor integration, Central Pattern Generators (CPGs), and morphological adaptation.
Meanwhile animals exhibit remarkable robustness to such disruptions, allowing them to recover and continue their tasks. Robots must achieve similar resilience, especially in high-stakes scenarios like search and rescue. My research, inspired by the robustness seen in animals, investigates enhancement strategies for robot locomotion through sensory-motor integration, Central Pattern Generators (CPGs), and morphological adaptation.
Why Are Animals Nailing It While Robots Keep Tripping Up?
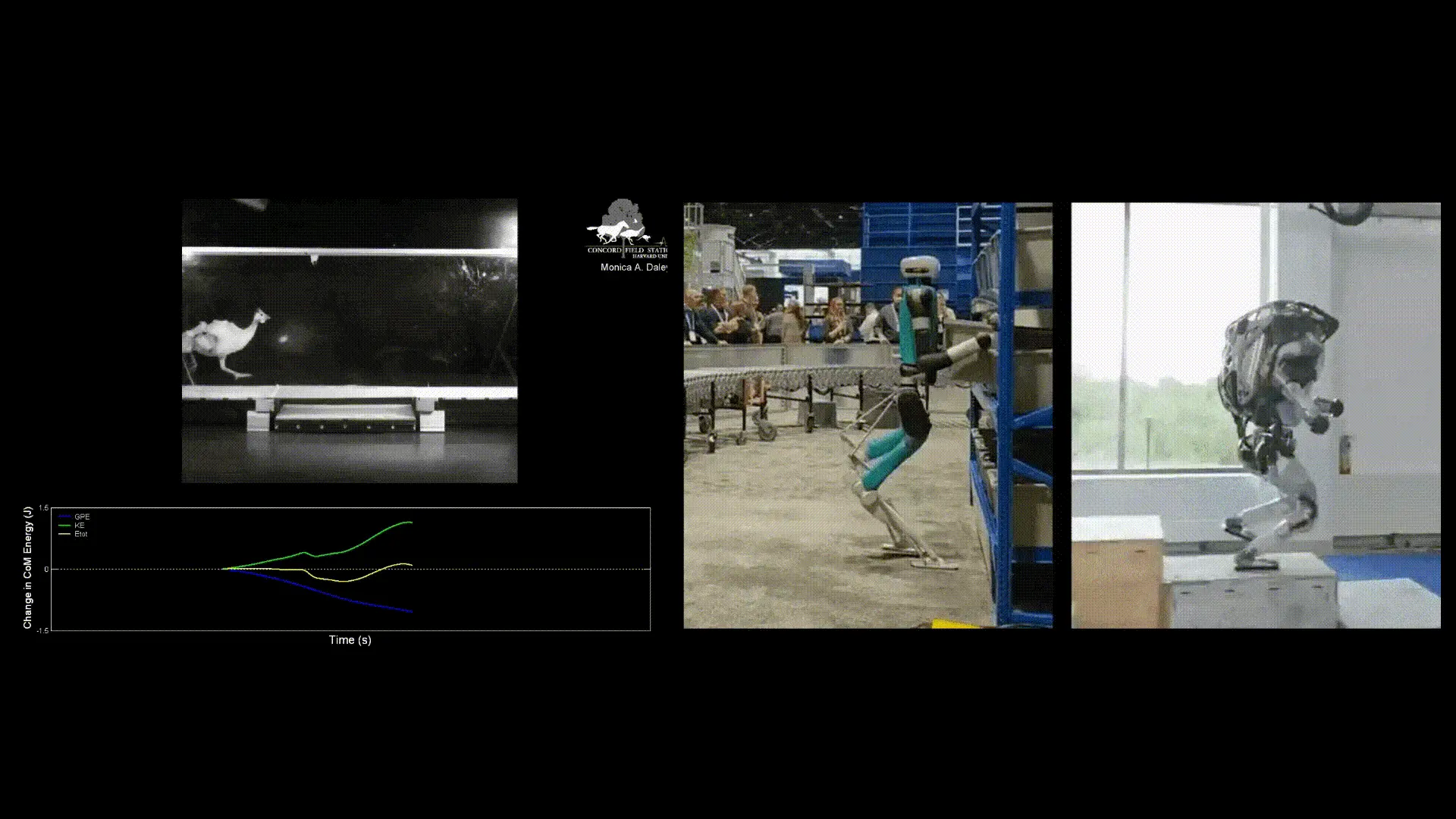
Running over rough terrain: guinea fowl maintain dynamic stability despite a large unexpected change in substrate height (Daley, Monica A., et al.)[left]. Agility Robotics's Digit [left], and Boston Dynamics's Atlas [right].
“Why Are Animals Nailing It While Robots Keep Tripping Up?” is a question that both roboticists and biologists are eager to answer. Animals benefit from sophisticated locomotor control, utilizing Central Pattern Generators (CPGs)—neural circuits in the spinal cord that generate rhythmic movement patterns—and higher brain centers that provide adaptive and goal-directed motor control. Their musculoskeletal systems offer dynamic flexibility and strength, enabling seamless adaptation to varying terrains. Moreover, animals possess acute sensory perception, integrating real-time environmental feedback to adjust movements instantly. By understanding and emulating these biological principles, robotics can improve and start to approach the performance levels seen in animals.
Illustration of different components of the locomotor system and their interaction in animals and bio-inspired robots. Mesencephalic Locomotor Region (MLR) is responsible for controlling locomotion initiation, speed and gait transitions. Reticulospinal (RS) neurons receive input from MLR and are responsible for controlling spinal locomotor circuits (CPG circuits).
To address the challenges in robot locomotion in unpredictable real-world environments, I am working on different projects that delves into various aspects of animal locomotion
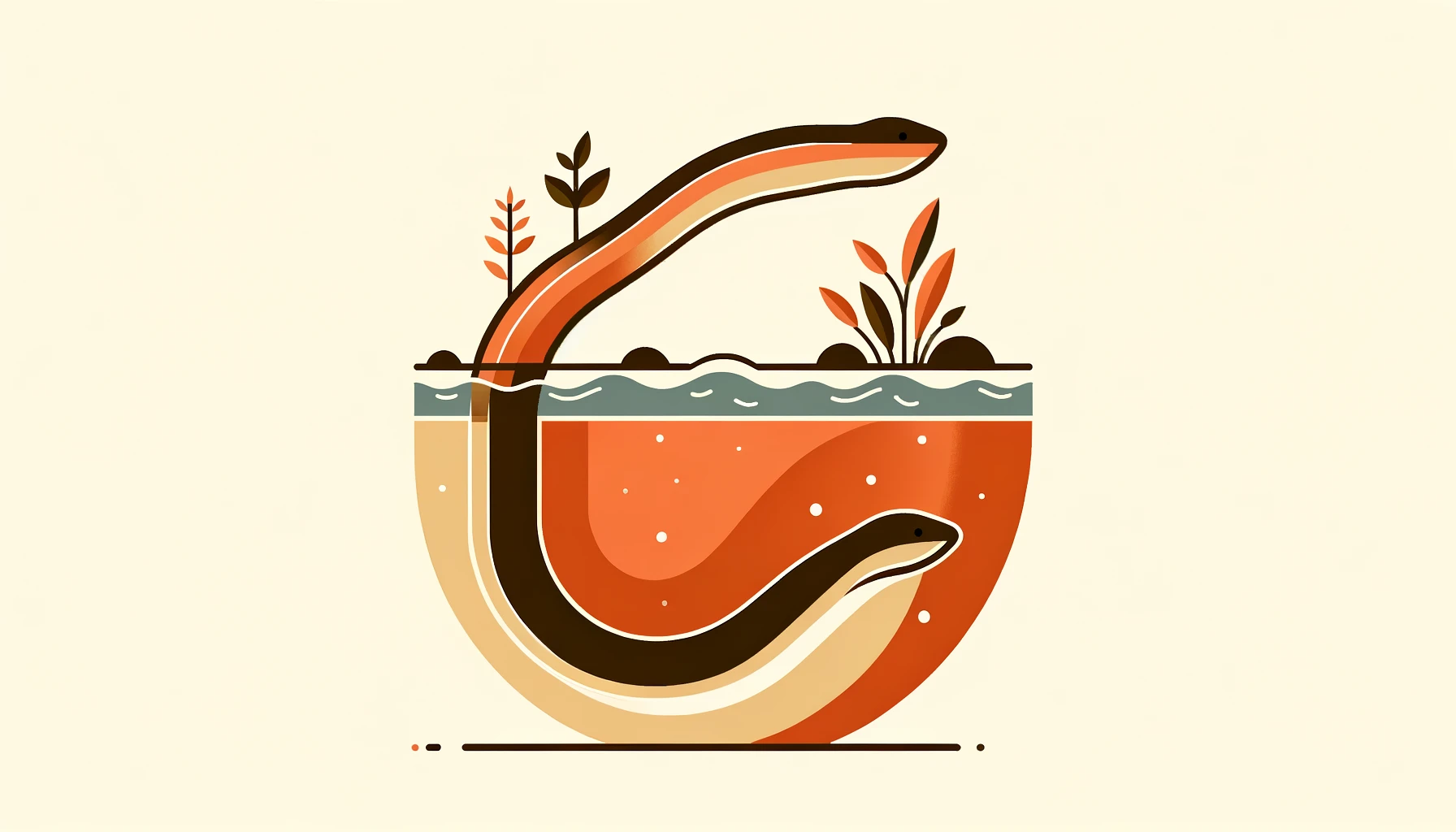
From Water to Land Unchanged
This project explores the transition of locomotion from aquatic to terrestrial environments in eel-like robots. By leveraging proprioceptive and somatosensory feedback, the study aims to develop multi-modal locomotion capabilities, mimicking the seamless movement observed in eels.
How Bio-Inspired Controllers Facilitate Sim-to-Real Transfer
This project examines the role of bio-inspired controllers in the sim-to-real transfer process. By drawing inspiration from biological systems, the research aims to develop controllers that can effectively bridge the gap between simulation and real-world application.
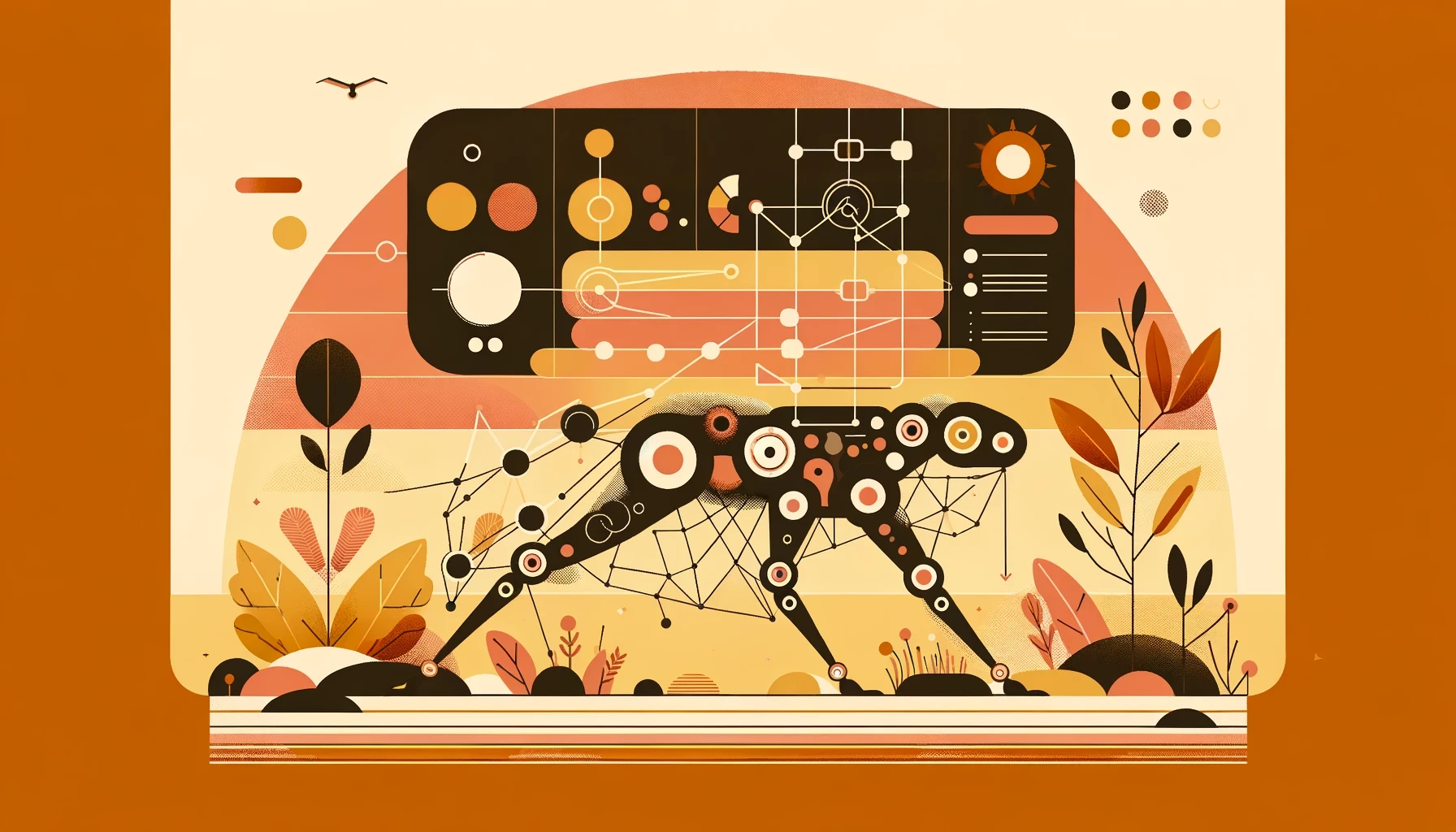
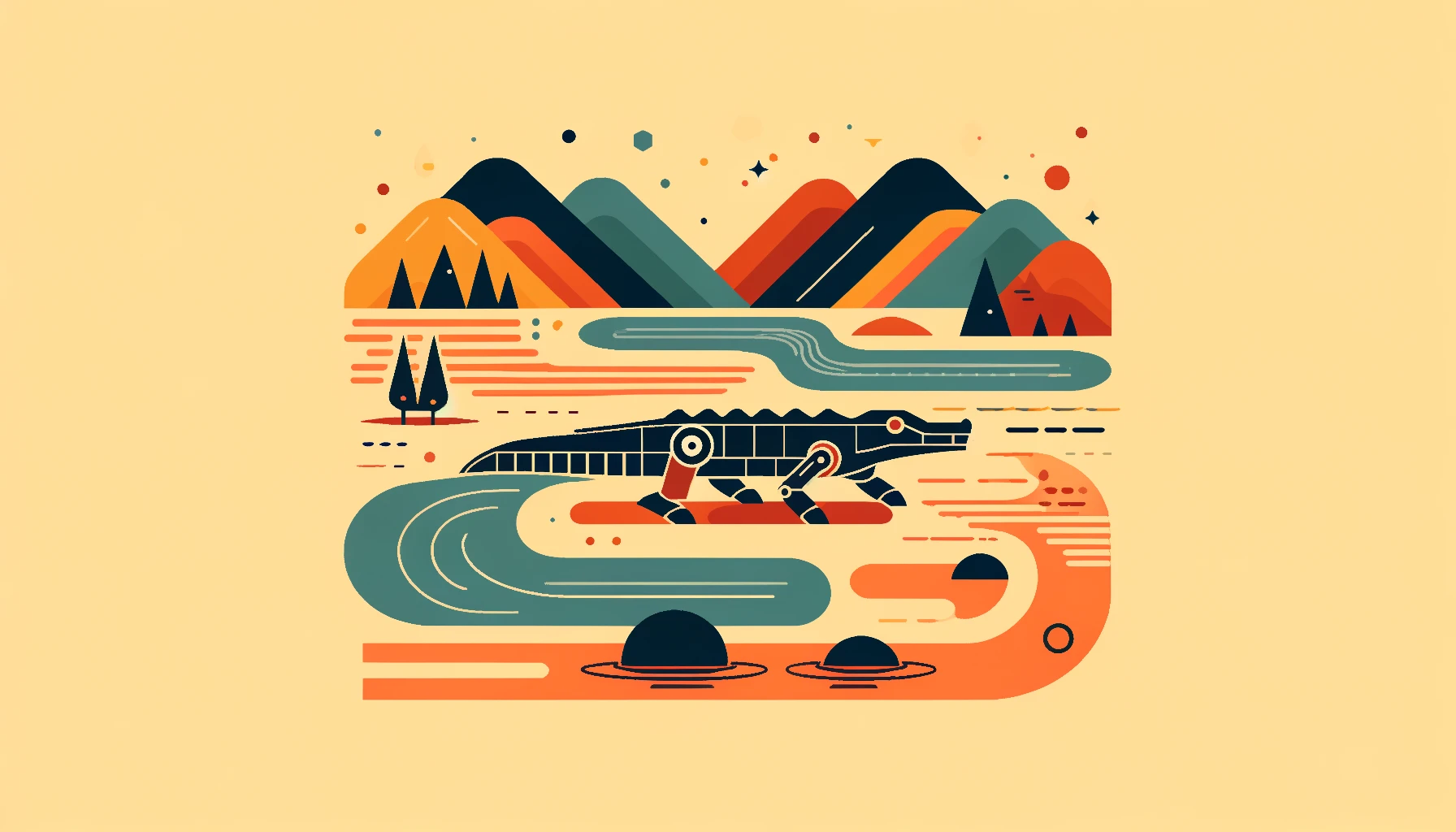
Amphibious Path Planning for Bio-Inspired Salamander-Like Robot
By leveraging deep learning, sophisticated path planning algorithms, and bio-inspired adaptive control mechanisms, the robot aims to efficiently navigate both aquatic and terrestrial environments. The primary objective is to enhance search and rescue operations, allowing the robot to seamlessly transition between different terrains, overcome obstacles, and perform critical tasks in complex, dynamic settings.
Deep Reinforcement Learning for Insights into Spatial, Topological, and Functional Integration of Proprioceptive Feedback during Undulatory Swimming
This research utilizes deep reinforcement learning to investigate how proprioceptive feedback integrates spatially, topologically, and functionally during undulatory swimming. By simulating these complex interactions, the project seeks to enhance our understanding of locomotor control in aquatic environments.
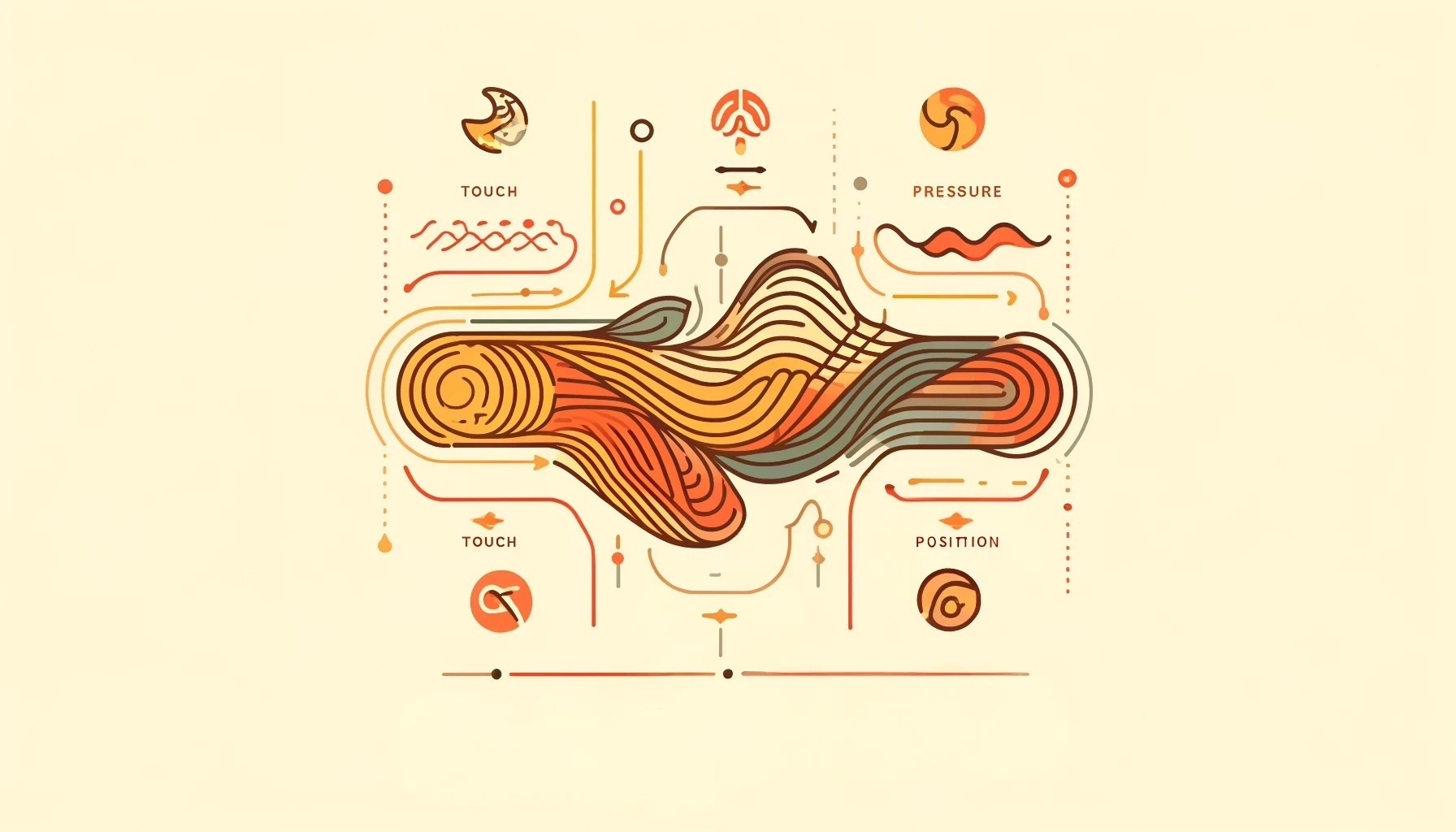